Automating face mask usage monitoring with Deep Learning
Considering the current evolution of the Covid-19 pandemic, face mask-wearing is likely to stay as a security measure for a while. Face masks are one of the best available preventive tools in closed public spaces, or in spaces where there are numerous people close next to each other.
What can organizations do to make sure that people comply with this safety mandate?
One option would be to make use of traditional methods such as direct observation by security agents, or manual, human monitoring of video feeds from security cameras. These are, however, time-consuming tasks that do not provide accurate results.
An alternative solution is Video Analytics using Deep Learning, which automates the process of monitoring and reporting face mask-wearing. This method is proven to deliver constant observation 24/7 with high accuracy and efficiency.
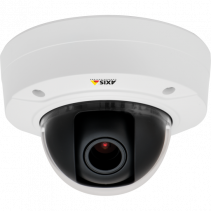
Using Deep Learning video intelligence, organizations can know in real-time, and also historically, whether people are wearing masks, and at which areas or timeframes the compliance percentage varies.
Maximizing the value of existing surveillance cameras
A major advantage of a Deep Learning Video Analytics system is that, in most cases, it is easily deployable on existing camera surveillance networks (or Video Management Systems – VMS). Organizations can make use of their current video surveillance assets, substantially cutting costs and saving time.
Configurable on an Intranet-based or on a Cloud-based architecture, the AI software takes upon the task of extracting, classifying and indexing the video streams coming from the cameras, and then displaying the data in the form of actionable and meaningful information that managers can use strategically.
Deep Learning Video Analytics engines use certain algorithms, patterns, and criteria to make sense of the video streams. They can be configured to identify as masks the various types commonly used: N95 masks, surgical masks, masks with various colors, or prints.
Additionally, by setting custom thresholds, managers can create triggers that alert when face mask usage is below a certain standard. This enables them and security agents to intervene quickly and determine a course of action to increase face mask-wearing if needed.
Using aggregated data for insightful decision-making
AI Video Analytics systems can aggregate data over time and display it on customized dashboards or reports in the form of diagrams, graphs, histograms, or heatmaps. These display trends with hourly, daily, or weekly granularity, and with geographical detail.
The power and value of statistical data are best used in combination with real-time alerts. Organizations can maximize their investments by employing the statistical data for determining thresholds, after which they set up custom alerts that notify security agents in real-time when irregularities occur. In this way, security personnel have a better situational awareness and faster response times.
The statistics become powerful strategic tools for managers to take safety and operational decisions. Managers can decide whether they need to take new measures to encourage face mask wearing. They can create a public awareness campaign to inform people about the importance of wearing masks, deploy additional security staff on-site, or, if available, hand out face masks.
Deep Learning Video Analytics are evolving into powerful applications that provide cost, efficiency, and automation advantages over the traditional video surveillance management systems. They are helping to streamline operational processes, save time, and create more accurate, strategic safety plans using the valuable insights from data.